Vol 7 No 1 2022- 1
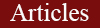
Predicting mortality in critically ill patients with COVID-19 in the ICU from a secondary-level hospital in Ecuador
Jorge Luis Vélez-Paez1,2*
, Mario Patricio Montalvo1, Fernando Esteban Jara1, Santiago Aguayo-Moscoso1, Wendy Tercero-Martínez1, Lenin Stalin Saltos1, Glenda Jiménez-Alulima1, Estefanía Irigoyen-Mogro1, Evelyn Castro-Reyes1, Christian Mora-Coello1, Edgar López-Rondón1, Patricio Toapanta1,2, Eduardo Vásconez-González3, Esteban Ortiz-Prado3

1. Intensive Care Unit – Pablo Arturo Suárez Hospital.
2. Faculty of Medicine, Universidad Central del Ecuador.
3. One Health Research Group, Faculty of Health Sciences, Medical School, Universidad de las Americas
* Corresponding Author:jlvelez@uce.edu.ec
Available from: http://dx.doi.org/10.21931/RB/2022.07.01.1
ABSTRACT
Since its molecular isolation on January 7, 2020, the new SARS-CoV-2 coronavirus has spread rapidly, affecting regions such as Latin America. Ecuador received the worst outbreak globally if we count excess mortality per capita. This study describes the clinical, epidemiological and therapeutic characteristics of 89 patients admitted to an intensive care unit (ICU) in a second-level hospital in Quito, Ecuador. Methods: We conducted a retrospective cohort study. We collected data from health records of adult patients with severe COVID-19 admitted to an ICU in Quito, Ecuador, during the first five months of the SARS-CoV-2 outbreak. We used the Chi-square test or Fisher’s exact statistics to analyze risk and associations between survivors and non-survivors. We used ROC curve analysis to predict mortality and determine cut-off points for mechanical, analytical, and cytometric ventilation parameters. We used the Wald test to evaluate the categorical predictors of the model at the multivariate level during the regression analysis. Results: 89 patients were recruited. The mean age of the patients was 54.72 years. Men represented 68.54% (n=61) and women 31.46% (n=28). Significant differences in mortality were observed (men 40.98% vs. women 17.76%). LDH and IL-6 at 24 hours after hospital admission were higher among non-survivors than survivors. Persistent hypercapnia (PaCO2 >45 mmHg), a PaFiO2 ratio of less than 140 mmHg, and positive end-expiratory pressure (PEEP) titration >9 mmHg were also associated with increased mortality. Conclusions: Elevated levels of LDH at 24 hours, IL-6 at 24 hours, lymphocyte and platelet count at 48 hours, neutrophil count at 48 hours and NLR are factors associated with higher motility, higher risk of failed extubation and reintubation in patients with acute respiratory distress syndrome due to COVID-19.
Keywords. COVID-19; ICU; ROC curve; Mortality; Low-Middle Income Country
INTRODUCTION
On 31 December, the Wuhan Municipal Health Committee informed the World Health Organization (WHO) that 27 people had been diagnosed with a type of pneumonia never described before1. On 7 January, 2020, Chinese scientists had isolated and sequenced the etiological agent, a novel beta coronavirus later identified as SARS-CoV-2 (severe acute respiratory syndrome coronavirus type -two)2. The genome of this RNA virus was made available on 12 January, 2020, allowing laboratories in different countries to produce specific primers for the infection diagnosis using real-time reverse transcription-polymerase chain reaction (RT-PCR) 2,3. On 11 March, 2020, the WHO declared COVID-19 a pandemic after the virus arrived in several countries rapidly4. Up to 5 October, 2020, more than 35 million people had been infected, causing more than 1 million deaths worldwide3.
In Latin America, a region with high levels of social inequality, mortality rates, and attack rate due to COVID-19, is devastating, especially for those living in poverty5. Households in the lowest income group have reduced access to health services, molecular diagnosis, and treatment. Health systems with scarce economic resources and disrupted contact tracing capabilities are often incapable of controlling outbreaks at the community level, affecting mortality and hospital admission trends6.
In mid-February 2020, the disease reached Latin America and hit Ecuador abruptly. The first case in Ecuador was officially reported on 27 February, but the only scientific report suggests that the virus has entered the country weeks earlier7. In March, the virus had spread massively within Ecuador’s coastal provinces, causing thousands of deaths each day in Ecuador, highlighting Guayaquil as the first COVID-19 epicenter in Latin America and the worst-hit country in the world8.
There is only one report exploring the epidemiological trends of COVID-19 in Ecuador, including a brief description of the clinical presentation among asymptomatic and mildly ill patients; nevertheless, no data is available in terms of the clinical features and outcome among critically ill patients7.
This study aims to present the outcome, and clinical characteristics of COVID-19 patients admitted to the intensive care unit in a secondary level hospital in Quito, Ecuador, from 1 April, 2020, to 31 July, 2020.
MATERIALS AND METHODS
Setting
The study was carried out in the Intensive Care Unit in the secondary level hospital Pablo Arturo Suárez Hospital, Quito, Ecuador. Quito is the capital of Ecuador and has a population of 2.781.641. The city is located in the province of Pichincha and has an elevation of 2,850 m above sea level, becoming the second-highest capital city globally.
Study design
A retrospective cohort study of the clinical course and mortality due to COVID-19 among adult patients hospitalized and admitted to the ICU from 1 April to 31 July, 2020
Population and sample size
Every patient admitted to the ICU with a suspected diagnosis of COVID-19 within the established timeframe was initially included in the study. At the end of the study, we included 89 patients that fulfilled the inclusion criteria, while 12 were excluded from the study.
Inclusion criteria
Every patient admitted to the ICU unit with a positive molecular, serological, or clinical diagnosis of COVID-19 (characterized by: fever or chills, cough, dyspnea, anosmia, ageusia, sore throat, or myalgia) was included in the study. The clinical records (HC) of patients admitted with a confirmed result of COVID-19 by RT-PCR [1] or suggestive tomographic pattern (CO-RADS 4 or 5) were included, and cases with a presumptive diagnosis of COVID-19.
Exclusion criteria
Patients with a mild clinical presentation that were not admitted to the ICU or those with respiratory symptomatology that tested negative for SARS-CoV-2 infection through molecular testing (RT-PCR) or tomographic pattern not compatible with COVID-19 (CO-RADS 0 to 3).
Variables and measurements
Our team reviewed the electronic records of every patient that fulfilled the inclusión criteria. (Being admitted to the ICU was one of them).[2] Information concerning epidemiological, clinical, serological, and cytometric variables was collected. Every record was reviewed, and data were retrieved from admission to discharge or death in the ICU during the data collection period.
Statistical analysis
We performed a complete descriptive statistical analysis, calculating every qualitative variable’s absolute and relative values. Mean, and standard deviation measures were used to describe differences and dispersion of the data set.
The assumption of normality of the quantitative variables was verified using the Shapiro test, where the t-test was used for parametric quantitative variables and the Mann Whitney test for those with non-parametric distributions.
We used the Chi-square test or Fisher’s exact statistic to compare the proportion of survivors and non-survivors due to COVID-19. An odds ratio greater than one was used to indicate that the outcome was more likely to occur in one group.
We used the ROC curve analysis to predict mortality, determining cut-off points using the Younden index for the parameters related to mechanical ventilation and cytometric parameters. At the multivariate level, the Wald method forward logistic procedure regression was used, determining predictors of mortality for COVID-19. Statistical significance was established for p-value <0.05.
Ethical considerations
According to human research’s local bioethical principles, anonymized, unidentifiable data from clinical records, excluding case reports, do not require internal review boards’ approval. The physicians involved in collecting clinical data were also the only health providers accessing patients’ clinical records.
RESULTS
General results
During 121 days of follow-up, 89 patients with COVID-19 fulfilled the inclusion criteria. 68,54 % (n=61) were men and 31,46% women (n=28). There was no statistically significant difference in mean length of hospital stay (ALOS) between those who survived (9.31 days) versus those who died (10.29 days). The follow-up ended with 66.29% of patients (n=59) discharged from the ICU unit, while 33.71% of them (n=30) died due to COVID-19 (Table 1).
Age and sex differences
The average age of patients admitted to the hospital was 54.7 years, and survivors were 11 years younger (50.9) than non-survivors (62.2), and this difference was statistically significant (p-value: 0.001). Regarding gender, men were three times more likely to die (40.98%, n = 25) from COVID-19 compared to women (17.76%, n = 5) (p-value: 0.032).
Comorbidities and mortality risk
The most frequent comorbidity reported was hypertension (HT) in 20.22% (n=18) followed by obesity 16.85% (n=15) and diabetes mellitus (DM) 8.99% (n=8). The mean body mass index (BMI) was 30.84, with significant differences being observed between survivors (28,98) and non-survivors (31.99), [5] being these differences statistically significant (p-value: 0.026).
Assessment of mortality indicators
The sequential organ failure assessment (SOFA) score at 24, 48, and 72 hours after admission was found to be 7.91, 6.14, and 5.46, respectively. The differences were statistically significant at 48 hours in survivors (SOFA=5.72) vs non-survivors (SOFA=7) and at 72 hours (SOFA= 4.93) versus (SOFA= 6.62) with a p-value of 0.038 and 0.010 respectively.
The APACHE II indicator was found to be higher among non-survivors (19.37) versus those who survived (17.42), although the differences were not statistically significant (p-value: 0.197) (Table 1).
Treatment and mortality
Seventy-two patients (80.9%) received systemic corticosteroid treatment ([7] dexamethasone 6 mg daily up to 10 days), and among those, 25 patients (34.72%) did not survive while 47 (65.28%) survived. Low molecular weight heparin (LMWH) was administered to 69 patients (78.41%), and from this group, 59.42% (n=41) survived, and 40.58% died (n=28).
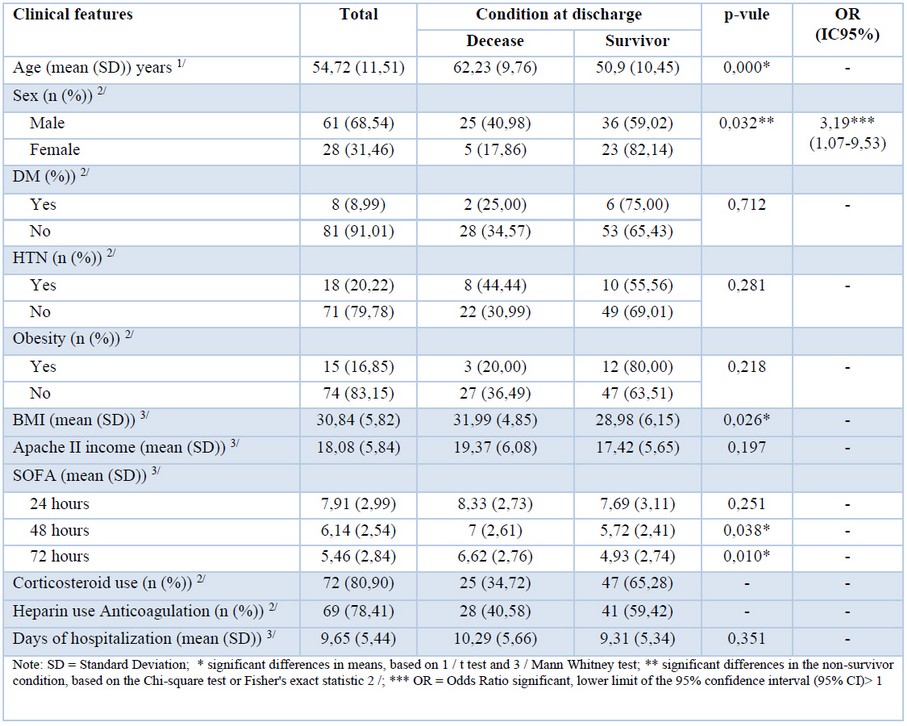
Table 1. Association between mortality and clinical characteristics.
Ventilatory and respiratory parameters
To mitigate end-expiratory alveolar collapse, applied extrinsic PEEP values at 48 hours were significantly lower (7.89 cmH2O) among survivors versus non-survivors (9.26 cmH2O), and this difference was statistically significant (p-value: 0.015). The maximum PCO2 at 72 hours was higher among non-survivors (49.34 mmHg), versus survivors (41.37 mmHg), and this difference was statistically significant (p-value: 0.026) (Table 2).
The PaO2/FiO2 ratio at 24 and 72 hours was always higher among survivors. For instance, non-survivors reported a PaO2/FiO2 of 127.77 mmHg and 136.36 mmHg at 24 and 72 hours, respectively, while survivors had values of 152.97 mmHg and 181.09 mmHg at the same time interval, both differences being statistically significant (p-value: 0.036 and <0.001 respectively).
Survivors remained intubated for seven days while non-survivors for ten days, a difference that is also statistically significant (p-value: 0.002).
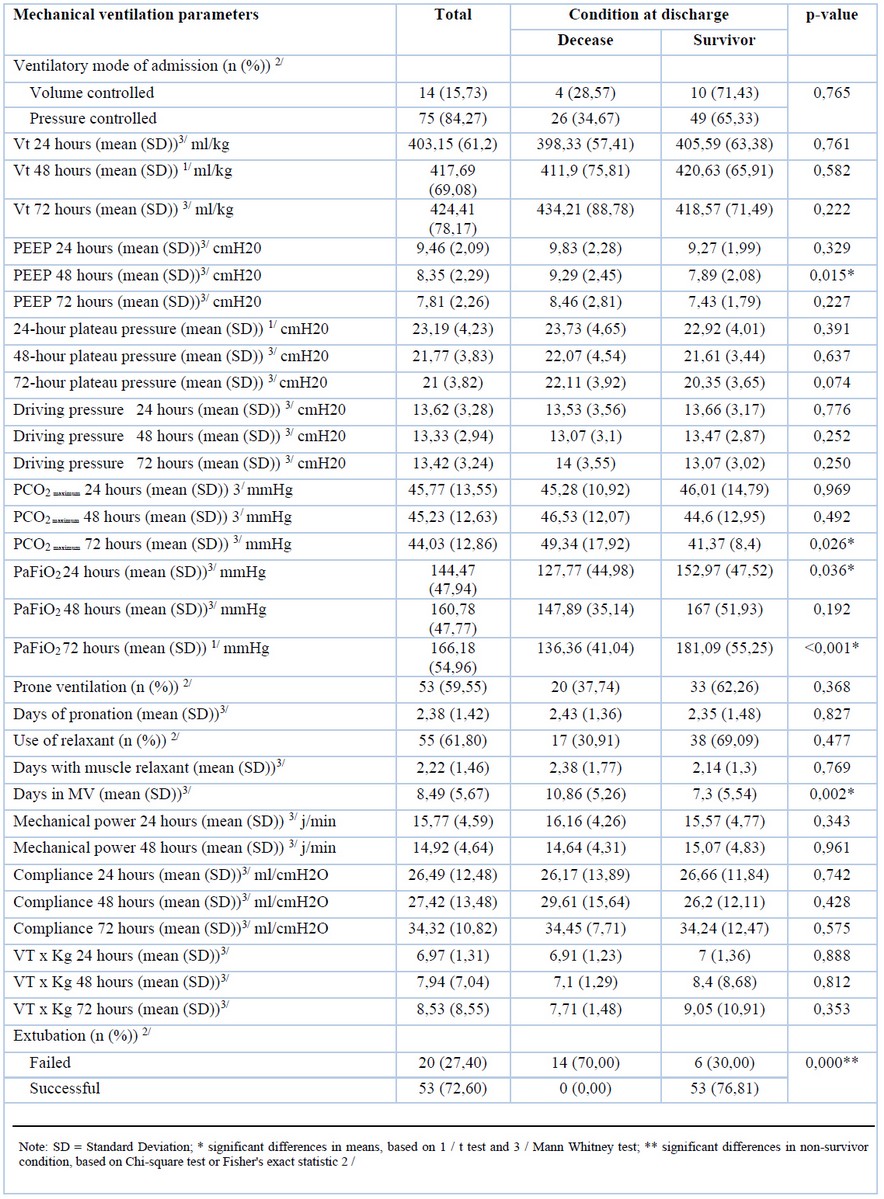
Table 2. Correlation between mortality and mechanical ventilation parameters.
Serological biomarkers
Mean lactate dehydrogenase levels (LDH) were higher among non-survivors (1025.47 U / L) versus survivors (891.10 U / L); Likewise, IL-6 presented was 137% higher among non-survivors (140.55 pg/ ml) versus survivors (59.3 pg. / ml) (p-value: < 0.05). D-dimer and ferritin at 24 and 48 hours did not show significant differences (Table 3).
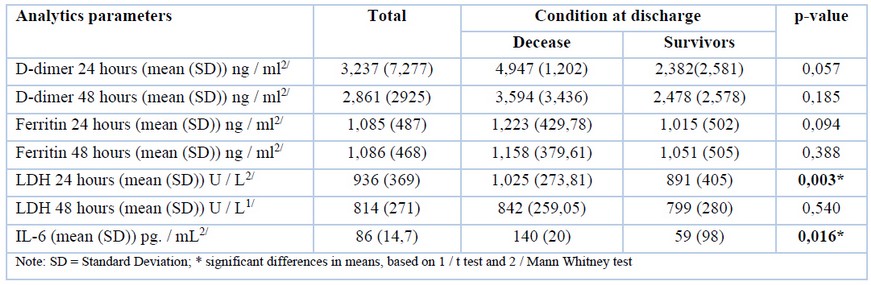
Table 3. Correlation between mortality and analytical parameters.
Flow cytometric analysis
Lymphocyte count at 48 hours presented a mean of 753.79 x 103 / ml in survivors and 537.59 x 103 / ml in non-survivors (p-value 0.006). Neutrophilia was found to be significantly higher among non-survivors at 24 hours (11,741.63 x 103 / ml) in comparison with survivors (9,282.54 x 103 / ml). For the neutrophil-lymphocyte ratio (NLR) [8] at 24, 48 and 72, non-survivors had significantly higher NLR than survivors (p-value: < 0.001) (Table 4).
Platelet count at 48 hours shows that non-survivors had significantly lower platelet counts (320,103.45 x 103 / ml) than survivors (388,172.41 x 103 / ml).
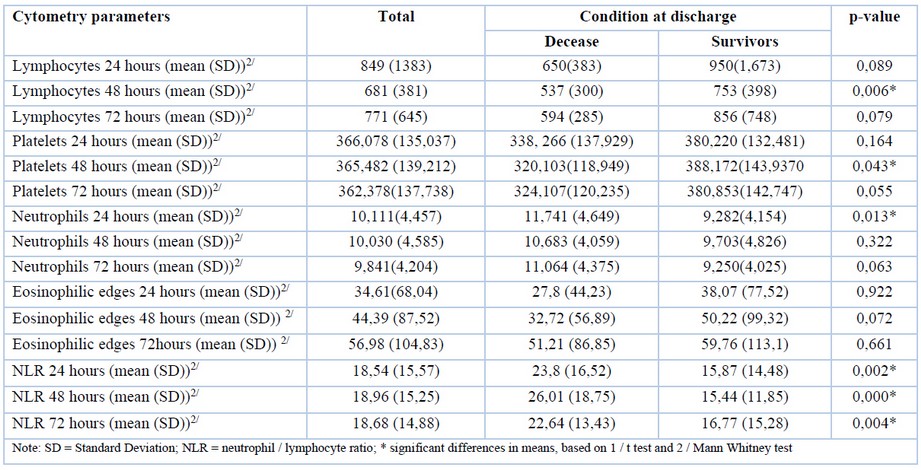
Table 4. Correlation between mortality and cytometric parameters.
Predictive factors for mortality
PEEP analysis.
The area of the receiver operating characteristic (ROC) curve for PEEP at 48 hours was 0.661 (95% CI 0.535-0.787), maximum PCO2 at 72 hours 0.650 (95% CI 0.519-0.780), PaFiO2 at 24 hours 0.636 (95% CI 0.508-0.765), and PaFiO2 at 72 hours 0.747 (95% CI 0.638-0.857), these areas presented confidence intervals that do not include the value 0.5; therefore, be used to predict mortality for COVID-19.
The cut-off points to predict mortality in the ROC curve using the Youden index of the mechanical ventilation parameters were positive for mortality if 48-hour PEEP ≥8.50 cmH20, where the sensitivity was 54%, and specificity was 74% (Figure 1).[9] Positive for mortality if: 72-hour peak PCO2 ≥46.50 mmHg (sensitivity: 54%, specificity 77%), 24-hour PaFiO2 ≤89 mmHg (sensitivity: 30%, specificity: 97%) and PaFiO2 72 hours ≤155.50 mmHg (sensitivity: 82%, specificity: 66%).
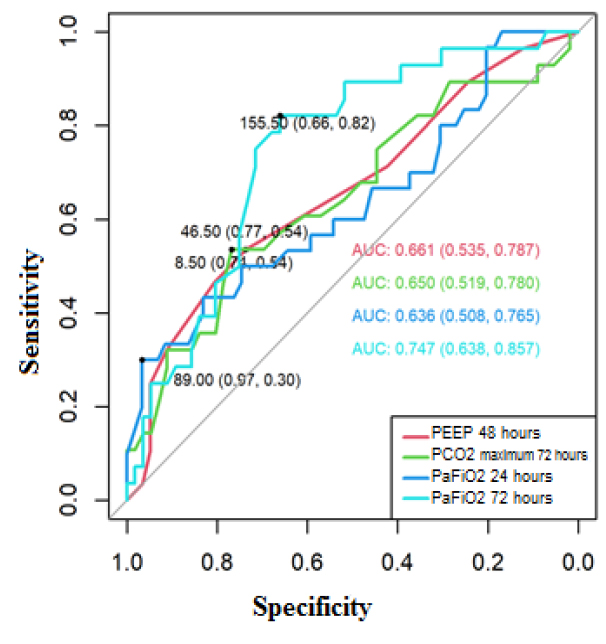
Figure 1. ROC curve to predict mortality for COVID-19, based on PEEP 48 hours, maximum PCO2 72 hours, PaFiO 2 24 and 72 hours.
Biomarker analysis.
The area of the ROC curve for IL-6 was 0.675 (IC95% 0.542-0.809), and for LDH at 24 hours 0.691 (IC95% 0.580-0.803), these areas presented confidence intervals that do not include the value 0.5; therefore, they are significant in predicting mortality for COVID-19.
The cut-off points to predict mortality in the ROC curve using the Youden index of the analytical parameters were positive for mortality if IL-6 ≥117 pg / mL, where the sensitivity was 42%, and specificity was 91%. Regarding LDH, it was positive for mortality at 24 hours with a cut-off≥783 U / L (sensitivity: 90%, specificity: 43%) (Figure 2).
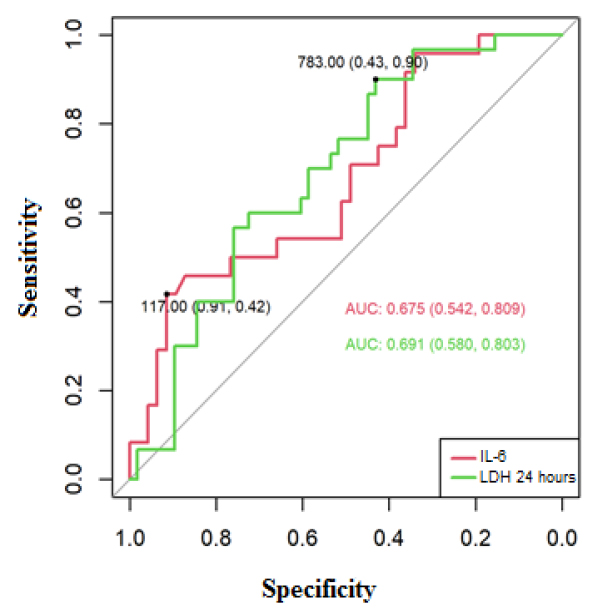
Figure 2. ROC curve to predict mortality for COVID-19, based on IL-6 and LDH at 24 hours.
The area of the ROC curve for NLR at 24 hours was 0.704 (95% CI 0.591-0.817), NLR at 48 hours[10] 0.743 (95% CI 0.634-0.851), NLR at 72 hours 0.692 (95% CI 0.578-0.806), and platelets 48 hours[11] 0.633 (95% CI 0.508-0.759), these areas presented confidence intervals that do not include the value 0.5; therefore, they are significant to predict mortality for COVID-19 (Figure 3).
The cut-off points for predicting mortality in the ROC curve using the Youden index of the cytometry parameters were the following: Positive for mortality if NLR 24 hours[13] ≥16.33, where sensitivity was 73% and specificity 64%. Positive for mortality if NLR 48 hours ≥16.96, where the sensitivity was 76% and specificity was 67%. Positive for mortality if NLR 72 hours ≥17.12, where the sensitivity was 64% and specificity 74%. Positive for mortality if Platelets ≤364,000 x 103 ml, where the sensitivity was 79% and specificity 50%.
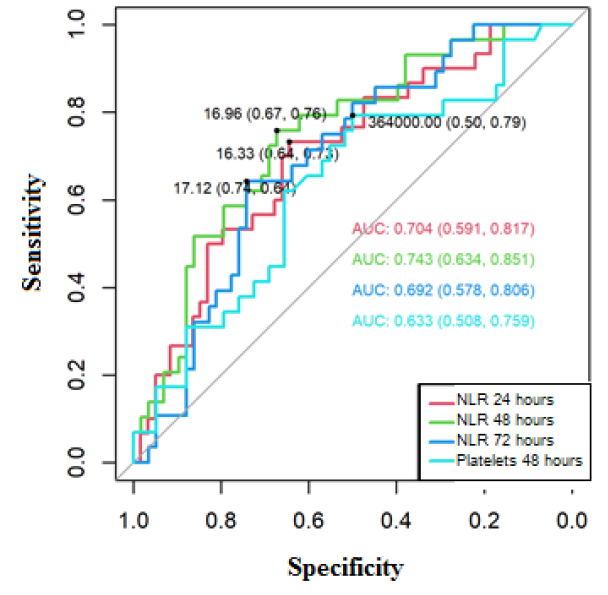
Figure 3. ROC curve to predict mortality for COVID-19, based on NLR (at 24, 48 and 72 hours[14] ) and platelets at 48 hours[16] .
SOFA mortality prediction analysis.
For the SOFA mortality predictors, the cut-off point for COVID-19 was determined, the ROC curves showed for SOFA at 48 hours an area of 0.637 (95% CI 0.511-0.763), and for 72 hours of 0.675 (95% CI 0.556 -0.794), these areas were significant to predict mortality, the cut-off point established at 48 and 72 hours was positive for mortality if SOFA≥6, at 48 hours, the sensitivity of 79% and specificity 48% were obtained, at 72 hours the sensitivity was 69% and specificity 57% (Figure 4).
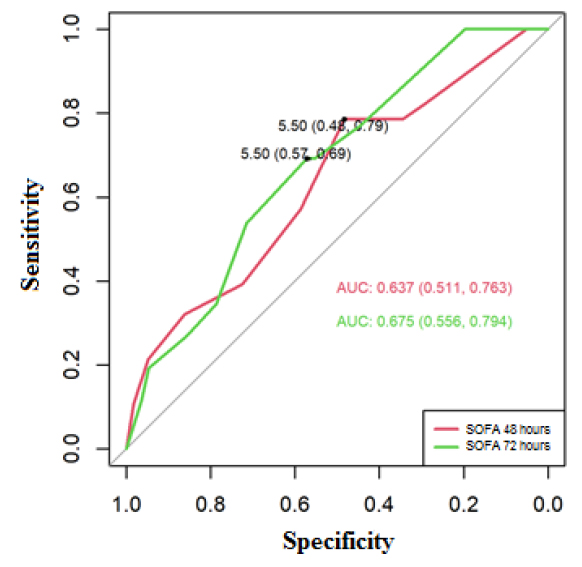
Figure 4. ROC curve to predict mortality for COVID-19, based on SOFA at 48 and 72 hours.
The results obtained showed that PaFiO2 72 hours ≤ 155.50 mmHg with p-value 0.009, IL-6 ≥117 pg. / mL with p-value 0.011, NLR 24 hours ≥16.33 with p-value 0.013 and NLR 72 hours ≥ 17.12 with p-value 0.005 are predictors of mortality for COVID-19; where values of PaFiO2 72 hours ≤ 155.50 mmHg, IL-6 ≥117 pg. / mL, NLR 24 hours ≥16.33 and NLR 72 hours ≥17.12 presented 9.24, 21.84, 6.13, and 13, 33 times more likely not to survive; the mechanical ventilation’s cut-off points, analytical and cytometry parameters were determined (Table 5).
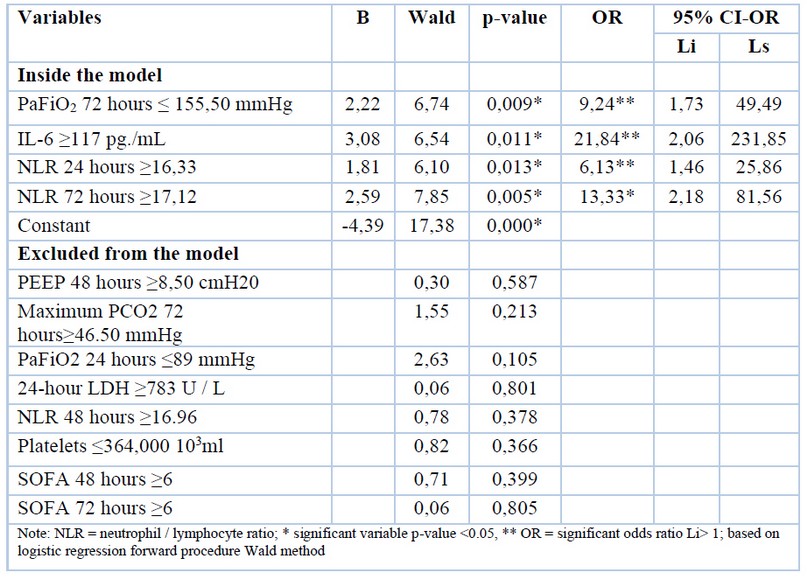
Table 5. Logistic regression to predict mortality for COVID-19, based on mechanical ventilation, analytical and cytometry parameters.
The results obtained showed that PaFiO2 72 hours ≤ 155.50 mmHg with p-value 0.009, IL-6 ≥117 pg. / mL with p-value 0.011, NLR 24 hours ≥16.33 with p-value 0.013 and NLR 72 hours ≥ 17.12 with p-value 0.005 are predictors of mortality for COVID-19; where values of PaFiO2 72 hours ≤ 155.50 mmHg, IL-6 ≥117 pg. / mL, NLR 24 hours ≥16.33 and NLR 72 hours ≥17.12 presented 9.24, 21.84, 6.13 and 13, 33 times more likely to not survive.
DISCUSSION
This original research is the first report of the clinical characteristics of severely ill patients with COVID-19 who have been clinically managed in a secondary-level hospital ICU in Quito, Ecuador. The results showed that older age and sex are positively associated with mortality. These results are similar to several reports available9,10. The average age of our admitted patients was 54 years, considerably younger populations than other countries. In China, two reports found that the mean age of patients admitted to the ICU was between 64 and 66 years, on average ten years older than our population11,12. In other continents, the age of the admitted patients is also higher. For instance, in Italy and Spain, the available information reports an average age of 63 years, while in the USA, the average age is 79 years10, 13-16.
Mejia et al. 2020 published the only available study with results similar to ours in a cohort of Peruvian patients. They found that the median age of the admitted patients was 59 years17. Although there is no clear information on why older men are at higher risk of dying due to COVID-19, a higher proportion of comorbidities among men may play a significant role, and the presence of unhealthier lifestyles[18] , along with immunosenescence phenomena. It has also been hypothesized that men the angiotensin-converting enzyme-2 (ACE-2) receptor might play an important role. Previously published studies suggest that the ACE-2 receptor plays a role in other coronaviruses-related diseases such as Severe Acute Respiratory Syndrome (SARS) or Middle East Respiratory Syndrome (MERS), finding higher concentrations of ACE-2 receptors among men18,19.
In terms of respiratory parameters, persisting hypercapnia for more than 72 hours, the PaFiO2 ratio at 24 and 72 hours < 140 mmHg and PEEP greater than 9 cmH2O were also associated with increased risk of mortality. External positive pressure ventilation increases intrathoracic pressure and does so more potently when the lungs are highly compliant 20. Moderate PEEP levels are required to ventilate adequately and achieve normoxia. In our results, maintaining PEEP levels greater than 8 mmHg after 48 hours was associated with a poorer prognosis. Although the impact of COVID-19 within the lungs is not quite the same as other diseases causing ARDS, the role of adequate ventilatory management is fundamental.
Gatinonni et al., defined two phenotypic patterns in the clinical presentation of COVID-19, a Low (L) phenotype in which there is low elastance, low shunt and poor recruit ability with little response to PEEP and a High (H) phenotype, with high elastance, high shunt and favorable response to alveolar recruitment with PEEP21,22.
Regarding the presentation of the L and H phenotypes in ARDS due to COVID 19, we consider that their presentation was variable, if we take into account the relationship between compliance and PaO2 / FiO2 as reported by Panwar23, patients with lower PaO2 / FiO2, like those with low compliance died. However, these variables can be very heterogeneous because there could be H patterns with PaO2 / FiO2 greater than 150 and in other L phenotypes with PaO2 /FiO2 <150mmHg. Both PaO2 / FiO2 and compliance have always been considered a marker of severity; in our work, the patients who had lower values were the most serious and of them, those who died had low compliance from admission, as mentioned. In other studies, patients that improved PaO2 / FiO2 and compliance pattern L had better survival22,23.
Our study also found that elevated levels of IL-6[19] , LDH at 24 hours, lymphopenia at 48 hours, neutrophilia at 24 hours, and high NLR from admission to 72 hours were also associated with more significant mortality. Previous works have evaluated this, which may indirectly indicate a reaction due to the massive inflammatory response or the cytokine storm constantly related with more severe clinical presentations24,25. These results are similar to those previously reported worldwide; however, it is interesting to note that ferritin and the D-dimer biomarker have not achieved enough statistical power to predict mortality12,26. Furthermore, our findings support the use of cytometric analysis that is often affordable and available in low-resource settings.
Several laboratory data are identified as predictors of severity and mortality in COVID-19, such as elevated D-dimer, lymphopenia, increased LDH, thrombocytopenia, increased C-reactive protein, elevated ferritin and IL-6, among others27-34. In our study, the factors associated with mortality were LDH values at 24 hours, IL-6, the lymphocyte and platelet count at 48 hours, the neutrophil count at 48 hours, and the NLR in all its measurements; the latter, together with IL-6, reached a predictive level. These results are consistent with the existing evidence in the world. Interestingly, D-dimer and ferritin at 24 and 48 hours did not present a significant association with mortality, a finding that contradicts the existing evidence at that time.
The most frequent comorbidities in our patients were: hypertension, obesity and diabetes mellitus (DM). For diabetes and hypertension, there was no statistically significant difference in terms of risk of mortality; nevertheless, when evaluating body mass index, higher BMI was associated with a greater risk of dying (Table 1), as described in other studies26. A clinical report from Wang et.al; showed statistically significant differences in terms of mortality among patients with chronic hypertension12. On the other hand, other studies found that hypertension was not an independent factor in increasing mortality, opposing hypercholesterolemia and DM10.
In general terms, the overall mortality in our center seems to be adequate compared to other countries. In Ecuador, we found that 33.7% of patients succumbed in the ICU unit due to COVID-19. A recently published report from China, including 517 patients, reported an overall mortality rate of 37.7%12,13. These numbers seem to be lower than other reports coming from Europe. For instance, In Italy, Grasselli et al., 2020 included 1,715 patients, and they found that the overall mortality was superior to 48% [10]. In Spain, a national cohort of 736 patients reported mortality rates greater than 42%14. On the other hand, information emerging from the USA shows that mortality was significantly lower in a cohort of 1,392 patients. They reported an overall mortality of 23.6%13.
In Latin America, reports are scarce. We found that in Peru, the overall mortality rate among severely ill COVID-19 patients was 32.4%35. However, in this study, cut-off points for serological biomarkers and mechanical ventilation variables analysis were not determined, which might give a more in-depth insight into our results.
At the beginning of the pandemic, corticosteroids’ use was controversial, and their use focused on quenching the so-called «cytokine storm»27,36-38. During the first few months of the outbreak, few scientific societies recommended using systemic corticosteroids to treat ARDS. Nevertheless, in our hospital, we adopted the SARS and MERS guidelines, which could be associated with our relatively low mortality rates compared to other centers22, 39-41.
Limitations
Our results came from an intensive care unit of 7 beds. Therefore, collecting a representative sample was more prolonged than in other centers. It is essential to point out that a molecular analysis for COVID-19 using RT-PCR was not always available in situ; therefore, the diagnosis was based on radiological and clinical suspicion, and the confirmatory molecular or serological confirmation sometimes arrived days later.
CONCLUSIONS
The values of LDH at 24 hours, IL-6 the lymphocyte and platelet count at 48 hours, the neutrophil count at 48 hours, and the NLR are factors associated with mortality and were even determining factors for failure in the extubation and reintubation.
The clinical and physiopathological presentation of COVID-19 suggested a strong activation of the pro-inflammatory response; this meant that in our hospital, even without solid evidence, we indicated corticoids, a measure that was later validated by RCTs and meta-analyses. This would essentially justify the non-elevated mortality in our series of patients.
Although analytical markers such as IL 6 and LDH are acceptable and well-known parameters for managing critical patients, their availability is not universal, and IL 6 is expensive. Finding a surrogate such as the NLR, which has a predictive value in its measurements at 24 and 72 hours that is not depreciable; makes the findings of this study relevant by providing a cost-effective instrument derived from the standard blood count to establish the risk of death and severity in COVID-19
Ethics approval and consent to participate
According to human research’s local bioethical principles, anonymized, unidentifiable data from clinical records, excluding case reports, do not require internal review boards’ approval. The physicians involved in collecting clinical data were also the only health providers accessing patients’ clinical records.
Availability of data and materials
The datasets used and analyzed during the current study are available from the corresponding author on reasonable request.
Competing interests
The authors declare no conflicts of interest.
Funding
This study did not receive any funding.
Authors’ contributions
JLV was entirely responsible for conceptualizing the study and directing the team when collecting information. He drafted the first version of the manuscript and reviewed the final version. MPM, FEJ, SAM, WTM, LS, GJ, EI, EC, and CM were responsible for collecting information from the ICU unit and contributing equally to the data analysis. EVG was responsible for completing the dataset and completing the first draft of the manuscript. EOP was responsible for critically reviewing the first draft, completing the manuscript’s final version, and critically reviewing the entire analytical process around data collection.
Acknowledgments
To all our collaborators, including medical, nursing and support staff who have spent months working day and night in the ICU without any proper resting, helping patients who are battling against COVID-19.
REFERENCES
1. International Society for, Infectious Diseases. PRO/AH/EDR> COVID-19 update (59): global, cruise ship, WHO. 2020. https://promedmail.org/promed-post/. Accessed 30 September 2020.
2. CDC. 2019-nCoV Respuestas a las preguntas más frecuentes | CDC. CDC. 2020. https://web.archive.org/web/20200318025404/https:/www.cdc.gov/coronavirus/2019-ncov/faq-sp.html. Accessed 30 Sep 2020.
3. Ortiz-Prado E, Simbaña-Rivera K, Gómez- Barreno L, Rubio-Neira M, Guaman LP, Kyriakidis NC, et al. Clinical, molecular, and epidemiological characterization of the SARS-CoV-2 virus and the Coronavirus Disease 2019 (COVID-19), a comprehensive literature review. Diagn Microbiol Infect Dis. 2020;98:115094.
4. OMS. Cronología de actuación ante el COVID-19 – OPS/OMS | Organización Panamericana de la Salud. https://www.paho.org/es/panama/cronologia-actuacion-ante-covid-19. Accessed 30 Sep 2020.
5. Ortiz-Prado E, Cevallos-Sierra G, Henriquez-Trujillo AR, Lowe R, Lister A. Covid-19 in Latin America. The BMJ; 2020. https://blogs.bmj.com/bmj/2020/08/13/covid-19-in-latin-america/. Accessed 3 Oct 2020.
6. Decerf B, Ferreira FH, Mahler DG, Sterck O. Lives and livelihoods: estimates of the global mortality and poverty effects of the Covid-19 pandemic. The World Bank; 2020.
7. Ortiz-Prado E, Diaz AM, Barreto A, Moyano C, Arcos V, Vasconez-Gonzalez E, et al. Epidemiological, socio-demographic and clinical features of the early phase of the COVID-19 epidemic in Ecuador. medRxiv. 2020.
8. Lima E, Vilela E, Peralta A, Rocha MG, Queiroz BL, Gonz\aaga MR, et al. Exploring excess mortality in Latin America in the context of covid pandemic: the cases of Brazil and Ecuador. 2020.
9. Palaiodimos L, Kokkinidis DG, Li W, Karamanis D, Ognibene J, Arora S, et al. Severe obesity, increasing age and male sex are independently associated with worse in-hospital outcomes, and higher in-hospital mortality, in a cohort of patients with COVID-19 in the Bronx, New York. Metabolism. 2020;108:154262.
10. Grasselli G, Greco M, Zanella A, Albano G, Antonelli M, Bellani G, et al. Risk Factors Associated With Mortality Among Patients With COVID-19 in Intensive Care Units in Lombardy, Italy. JAMA Intern Med. 2020. doi:10.1001/jamainternmed.2020.3539.
11. Gao Q, Hu Y, Dai Z, Wu J, Xiao F, Wang J. The epidemiological characteristics of 2019 novel coronavirus diseases (COVID-19) in Jingmen,Hubei,China. medRxiv. 2020;:2020.03.07.20031393.
12. Wang Y, Lu X, Li Y, Chen H, Chen T, Su N, et al. Clinical Course and Outcomes of 344 Intensive Care Patients with COVID-19. Am J Respir Crit Care Med. 2020;201:1430–4.
13. Quah P, Li A, Phua J. Mortality rates of patients with COVID-19 in the intensive care unit: a systematic review of the emerging literature. Crit Care. 2020;24. doi:10.1186/s13054-020-03006-1.
14. Berenguer J, Ryan P, Rodríguez-Baño J, Jarrín I, Carratalà J, Pachón J, et al. Characteristics and predictors of death among 4035 consecutively hospitalized patients with COVID-19 in Spain. Clin Microbiol Infect. 2020;0. doi:10.1016/j.cmi.2020.07.024.
15. Richardson S, Hirsch JS, Narasimhan M, Crawford JM, McGinn T, Davidson KW, et al. Presenting Characteristics, Comorbidities, and Outcomes Among 5700 Patients Hospitalized With COVID-19 in the New York City Area. JAMA. 2020;323:2052–9.
16. Barrasa H, Rello J, Tejada S, Martín A, Balziskueta G, Vinuesa C, et al. SARS-CoV-2 in Spanish Intensive Care Units: Early experience with 15-day survival in Vitoria. Anaesth Crit Care Pain Med. 2020. doi:10.1016/j.accpm.2020.04.001.
17. Mejía F, Medina C, Cornejo E, Morello E, Vásquez S, Alave J, et al. Características clínicas y factores asociados a mortalidad en pacientes adultos hospitalizados por COVID-19 en un hospital público de Lima, Perú. 2020. doi:10.1590/SciELOPreprints.858.
18. Scully EP, Haverfield J, Ursin RL, Tannenbaum C, Klein SL. Considering how biological sex impacts immune responses and COVID-19 outcomes. Nat Rev Immunol. 2020;:1–6.
19. Sama IE, Ravera A, Santema BT, van Goor H, Ter Maaten JM, Cleland JG, et al. Circulating plasma concentrations of angiotensin-converting enzyme 2 in men and women with heart failure and effects of renin–angiotensin–aldosterone inhibitors. Eur Heart J. 2020;41:1810–1817.
20. Jardin F, Genevray B, Brun-Ney D, Bourdarias J-P. Influence of lung and chest wall compliances on transmission of airway pressure to the pleural space in critically ill patients. Chest. 1985;88:653–658.
21. Gattinoni L, Chiumello D, Caironi P, Busana M, Romitti F, Brazzi L, et al. COVID-19 pneumonia: different respiratory treatments for different phenotypes? Springer; 2020.
22. Montenegro F, Unigarro L, Paredes G, Moya T, Romero A, Torres L, et al. Acute Respiratory Distress Syndrome (ARDS) Caused by the Novel Coronavirus Disease (COVID-19): A Practical Comprehensive Literature Review. 2020.
23. Panwar R, Madotto F, Laffey JG, Van Haren FMP. Compliance Phenotypes in Early ARDS Before the COVID-19 Pandemic. Am J Respir Crit Care Med. 2020. doi:10.1164/rccm.202005-2046OC.
24. Sun X, Wang T, Cai D, Hu Z, Liao H, Zhi L, et al. Cytokine storm intervention in the early stages of COVID-19 pneumonia. Cytokine Growth Factor Rev. 2020.
25. Coperchini F, Chiovato L, Croce L, Magri F, Rotondi M. The cytokine storm in COVID-19: an overview of the involvement of the chemokine/chemokine-receptor system. Cytokine Growth Factor Rev. 2020.
26. Palaiodimos L, Kokkinidis DG, Li W, Karamanis D, Ognibene J, Arora S, et al. Severe obesity, increasing age and male sex are independently associated with worse in-hospital outcomes, and higher in-hospital mortality, in a cohort of patients with COVID-19 in the Bronx, New York. Metabolism. 2020;108:154262.
27. CDC. Coronavirus Disease 2019 (COVID-19). Centers for Disease Control and Prevention. 2020. https://www.cdc.gov/coronavirus/2019-ncov/hcp/clinical-guidance-management-patients.html. Accessed 30 Sep 2020.
28. Ministerio de Sanidad. INFORMACIÓN CIENTÍFICA-TÉCNICA Enfermedad por coronavirus, COVID-19. 2020. https://www.mscbs.gob.es/profesionales/saludPublica/ccayes/alertasActual/nCov/documentos/ITCoronavirus.pdf. Accessed 30 Sep 2020.
29. BMJ. Coronavirus disease 2019 (COVID-19) – Symptoms, diagnosis and treatment | BMJ Best Practice US. BMJ Best Practice. 2020. https://bestpractice.bmj.com/topics/en-us/3000168. Accessed 30 September 2020.
30. McIntosh K. Coronavirus disease 2019 (COVID-19): Epidemiology, virology, and prevention – UpToDate. UpToDate Inc. 2020. https://www.uptodate.com/contents/coronavirus-disease-2019-covid-19-epidemiology-virology-and-prevention. Accessed 30 September 2020.
31. Cochrane. El valor D-dímero está asociado a la gravedad de los pacientes con la COVID-19. cochrane.org. 2020. /es/recursos/evidencias-covid-19/el-valor-d-d%C3%ADmero-est%C3%A1-asociado-la-gravedad-de-los-pacientes-con-la. Accessed 30 Sep 2020.
32. Lippi G, Favaloro EJ. D-dimer is Associated with Severity of Coronavirus Disease 2019: A Pooled Analysis. Thromb Haemost. 2020;120:876–8.
33. Nasiri MJ, Haddadi S, Tahvildari A, Farsi Y, Arbabi M, Hasanzadeh S, et al. COVID-19 Clinical Characteristics, and Sex-Specific Risk of Mortality: Systematic Review and Meta-Analysis. Front Med. 2020;7. doi:10.3389/fmed.2020.00459.
34. Xu L, Yaqian M, Chen G. Risk factors for severe corona virus disease 2019 (COVID-19) patients : a systematic review and meta analysis. medRxiv. 2020;:2020.03.30.20047415.
35. Benitez H, Vargaas E, Peña E, Taype A, Arrospide D, Castillo M, et al. Características clínicas, manejo y mortalidad de pacientes hospitalizados con COVID-19 en un hospital de referencia en Lima, Perú. PRE-PRINT. 2020.
36. WHO. Clinical management of COVID-19. WHO. 2020. https://www.who.int/publications-detail-redirect/clinical-management-of-covid-19. Accessed 30 September 2020.
37. Ministerios de Sanidad. Protocolo_manejo_clinico_ah_COVID-19.pdf. 2020. https://www.mscbs.gob.es/profesionales/saludPublica/ccayes/alertasActual/nCov/documentos/Protocolo_manejo_clinico_ah_COVID-19.pdf. Accessed 30 Sep 2020.
38. Ministerio de Sanidad. Manejo_urgencias_pacientes_con_COVID-19.pdf. 2020. https://www.mscbs.gob.es/profesionales/saludPublica/ccayes/alertasActual/nCov/documentos/Manejo_urgencias_pacientes_con_COVID-19.pdf. Accessed 30 Sep 2020.
39. Anesi G. Coronavirus disease 2019 (COVID-19): Critical care and airway management issues – UpToDate. UpToDate Inc. 2020. https://www.uptodate.com/contents/coronavirus-disease-2019-covid-19-critical-care-and-airway-management-issues?search=coronavirus-disease-2019-covid-19-%20critical-care-issues%5D&source=search_result&selectedTitle=1~150&usage_type=default&display_rank=1. Accessed 30 September 2020.
40. Alhazzani W, Møller MH, Arabi YM, Loeb M, Gong MN, Fan E, et al. Surviving Sepsis Campaign: guidelines on the management of critically ill adults with Coronavirus Disease 2019 (COVID-19). Intensive Care Med. 2020;46:854–87.
41. Wu C, Chen X, Cai Y, Xia J, Zhou X, Xu S, et al. Risk Factors Associated With Acute Respiratory Distress Syndrome and Death in Patients With Coronavirus Disease 2019 Pneumonia in Wuhan, China. JAMA Intern Med. 2020;180:934.
Received: 15 September 2021 / Revised: 7 December 2021 / Accepted: 15 July 2022 / Published:15 February 2022
Citation: Vélez-Paez JL, Montalvo MP, Esteban Jara F , Aguayo-Moscoso S , Tercero-Martínez W , Stalin Saltos L , Jiménez-Alulima G , Irigoyen-Mogro E , Castro-Reyes E, Mora-Coello C , López-Rondón E, Toapanta P, Vásconez-González E , Ortiz-Prado E . Predicting mortality in critically ill patients with COVID-19 in the ICU from a secondary-level hospital in Ecuador. Revis Bionatura 2022;7(1) 1. http://dx.doi.org/10.21931/RB/2022.07.01.1