Vol 2 No 3 2017 – 13
NOTICIAS Y OPINIONES TÉCNICAS / TECHNICAL NEWS AND VIEWS
DNA microarrays: Recent Advances
Microarreglos de ADN: Avances Recientes
Henry J. Herrera1 – Marlon Gancino1
http://dx.doi.org/10.21931/RB/2017.02.03.13
_______________________________________________________________________________________________________________________
ABSTRACT
The present work shows a bibliographical compilation about DNA microarray technology. First, a brief summary is presented with the most important historical aspects that gave rise to this technology in the field of biotechnological research, until it became one of the most significant tools in the field of medical diagnosis. The concept of DNA microarray is clearly defined and an important emphasis is made on the main variants within this technology: oligonucleotide microarray and cDNA microarray. Subsequently, the major differences between the two DNA microarray classes are mentioned to culminate showing the advantages and disadvantages that each one has when it is time of a gene expression analysis. Furthermore, some applications that this technology offers in the biological-clinical field are cited. Finally, this work indicates the latest technological advances in data analysis from a bioinformatic approach and a pithy explanation is made about the use and operation of microarray databases.
Key words: cDNA microarray, oligonucleotide microarray, microarray applications, microarray analysis, microarray databases
_______________________________________________________________________________________________________________________
RESUMEN
El presente artículo es el resultado de una compilación bibliográfica acerca de la tecnología de microarreglos de ADN. Primero, se realiza una breve recapitulación de los aspectos históricos más importantes que dieron paso a esta tecnología en el campo de investigación biotecnológica, hasta llegar a ser una de las herramientas con mayor significancia en el campo de diagnóstico médico. Se define claramente el concepto de microarreglo de ADN y se hace un fuerte énfasis en las principales variantes dentro de esta tecnología: microarreglos de ADNc y microarreglos de oligonucleótidos. Ulteriormente, se mencionan las mayores diferencias entre las dos clases de microarreglos de ADN, para culminar mostrando las ventajas y desventajas que ambos tienen a la hora de realizar un análisis de expresión génica. Además, se citan algunas aplicaciones que esta tecnología ofrece en el campo biológico-clínico. Finalmente, se indica los avances tecnológicos más recientes en el análisis de datos desde una perspectiva bioinformática y se realiza una breve explicación acerca del funcionamiento y uso de bases de datos de microarreglos.
Palabras claves: microarreglo de ADNc, microarreglo de oligonucleótidos, aplicaciones de microarreglos, análisis de datos de microarreglos, base de datos de microarreglos.
_______________________________________________________________________________________________________________________
INTRODUCTION
The publication of the complete decoding of the human genome by The Human Genome Project (HGP) in 2003 1 brought to light the latent complexity that encompasses gene interaction in the phenotypic expression of each human being. Where, to control the necessary cellular mechanisms that mediate the transformation of a fertilized ovule into a mature human body and then keep it alive requires the intervention of every single gene 2. Each one of these regulated in a unique and precise way in relation to its location and its degree of expressivity so that together and working in a harmonious way give as final result life. Because of this, and in order to have a better understanding of the development of organisms, factors involved in the onset of genetic diseases, or simply to monitor the fluctuating expression of a single gene on a cell under specific conditions, it became imperative to create tools that allow efficient registration of the interactions between the basic macromolecules that regulate each vital cycle 3.
Classically, the techniques or tools that were used in the laboratory to perform the activities of gene monitoring were Southern and Northern blotting but because of the digitization of research tools in the last two decades these techniques were replaced by microarray technology 4. The introduction of this new tool in the biotechnology field allowed a much more efficient quantitative and simultaneous monitoring of the expression of thousands of genes 5. Being DNA microarray the most significant, important and best developed technology of all types of microarrays 6.
The development of DNA microarray technology in 1995 was founded with the refinement of the technique for the formation in-situ of oligonucleotides called photolithography and the creation of the first cDNA library in 1992 and 1994 respectively 6. In 1997 the first quantitative and simultaneous monitoring of the gene expression in one in a specific class of yeast, Saccharomyces cerevisiae, was obtained 7. The improvement in the efficiency of this technique allowed in 2003 to be introduced in the clinical field with a focus on improving the techniques of medical diagnosis 8. Finally, in 2004 this technique allowed to study the gene expression of the entire human genome in a single layer of DNA microarrays 9.
As evidenced in the previous paragraph, this is a technology that has undergone significant advances in terms of its refinement that have been relatively fast and whose theoretical foundation is essentially based on macromolecular complementarity 5. In a more detailed way, a DNA microarray works through a mechanism that is composed of the following elements: a) target: which, in DNA microarray, is specifically a defined sample of DNA previously marked immersed in a solution; b) labels: these are markers placed in the target DNA samples that when they undergo a change in their biochemical medium reacts by showing a specific color or a specific wavelength; c) probes: they are DNA sequences corresponding to specific genes placed on a solid support which will serve to determine the degree of gene expression exhibited by a particular cell; and d) a solid support, which is the place where DNA probe sequences are anchored 10. Thousands of slits are placed in a rectangular holder where each contains many pieces of DNA or DNA probe 3. The mRNA is extracted from a sample cell and the reverse transcription process is performed with the reverse transcriptase enzyme to obtain cDNA, thereby indicating which gene or which gene set is highly expressed by the mRNA of the cell sample 11. Each cDNA copy is labeled with a fluorescent or radioactive label type. The amount of hybridization that a cDNA sample displays with a specific plate of DNA microarrays is measured by scanning the wavelength or color change that each spot of the backing plate presents 12. In this way, this process can be summarized as the set of 5 experimental steps 13, which are: 1) biological query; 2) preparation of the sample; 3) biological reaction; 4) data visualization; and 5) modeling.
DNA microarray overview
DNA microarray could be defined in two ways, as an arrangement of known sequenced genes printed on a solid support e.g. glass microscope slides, silicon chips, plastics, nylon membrane, as well as a technology used to analyze and detect gene expression and mutations respectively 14. DNA microarray makes possible to identify genes that are being expressed due to changes in environmental parameters, cellular differentiation and mutations in metabolic pathways. Therefore, this technology is considered as an effective tool for genome-wide expression profiling 15. DNA arrays are printed with DNA, cDNAs, oligonucleotides (synthetic) or PCR products that normally represent a gene; an important advantage of DNA microarray is that it allows to study hundreds to thousands of genes at the same time, even an entire genome of an organism 14.
cDNA microarray vs oligonucleotide microarray
There two types of DNA microarrays, cDNA microarrays and oligonucleotide microarrays 5. Both microarrays are based on base complementarity and show the abundance of transcripts; usually two mRNA samples are prepared, the control and experimental sample, these mRNA samples are converted into cDNAs with reverse transcriptase 16 and can be labeled with fluorophore, silver, chemiluminescence 17. In the case of cDNA microarrays each sample is prepared with a different label and then both samples are mixed to finally be spotted in the microarrays where probes are ready to hybridize complementary sequences as in Figure 1. On the other hand, in oligonucleotide arrays, both samples use the same label, but the labeled cDNAs of each sample are hybridized in separate arrays 16 as in Figure 2.
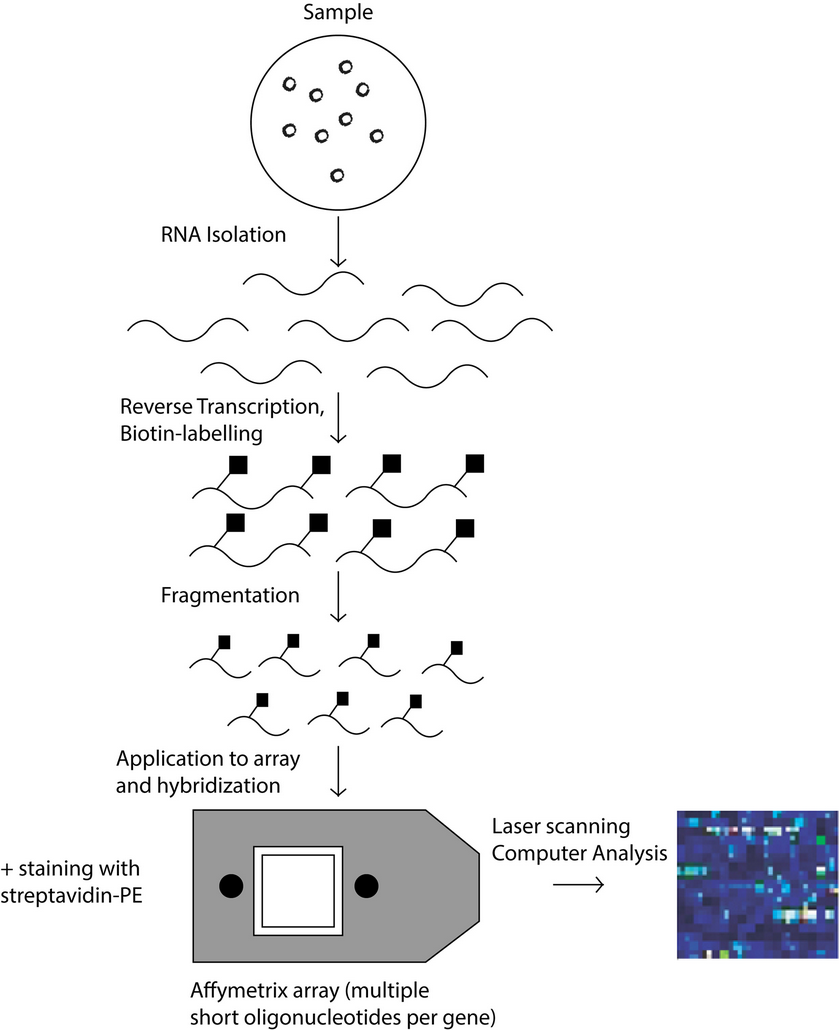
Figure 1: Schematic process of the operation of cDNA microarray technology.
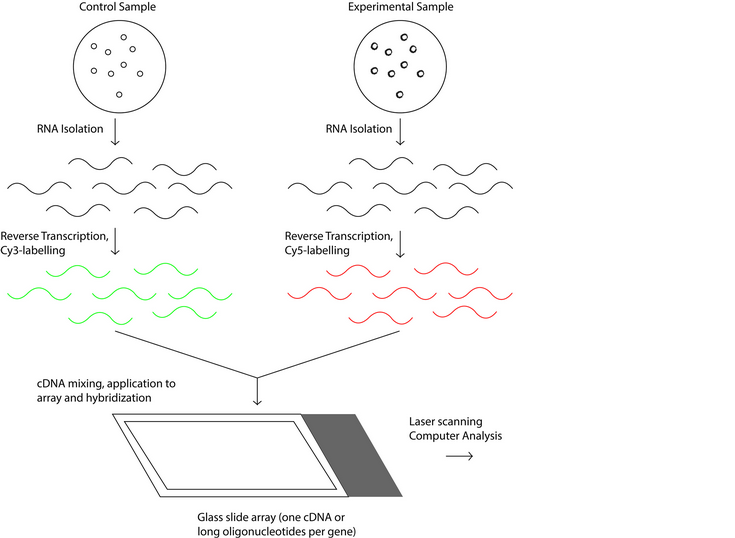
Figure 2: Schematic process of the operation of oligonucleotide microarray technology.
There are other differences between cDNA microarrays and oligonucleotide microarrays. In this last one, probes are printed or synthesized in situ usually by photolithographic deposition –the quality of the DNA chip depends on this technique- while in cDNA microarrays the probes are pre-fabricated and then robotically printed on the glass slide 16. Another characteristic to take in consideration is that oligonucleotides arrays contain probes with short fragments of DNA of about 25 base pairs while cDNA microarrays probes have long fragments of DNA from hundreds to thousands of base pairs 5. Because this technology has been advancing considerably these last years, many DNA microarrays with different characteristics (e.g. longer probes, RNA probes, DNA probes, improved microelectrode arrays, higher resolution) are available in the market and these are some of the commercial arrayers: Affymetrix, Invitrogen, Genome systems, Silicon genetics Biodiscovery, Genetix, Xeno 5.
The use of a specific type of DNA microarray is going to depend on what the investigation is about, but also on the resources available for it. That is why the review will show some advantages and disadvantages of each type of DNA microarray. cDNA microarrays have better detection sensitivity (probes are longer), a lower cost, and do not require specific equipment; actually, most of the equipment is often available in the laboratory. However, the time required to synthesize, purify and store DNA solutions before the fabrication of the microarray, along with more printing devices required and cross hybridization comprise the disadvantages of cDNA microarrays. On the other hand, oligonucleotide microarrays have some benefits too such as faster generation of the array, better specificity, less contamination and reproducibility. Not having to deal with the preparation and correct handling of probes (PCR products, cDNAs) reduce time, contamination and the possibility of mix up. The design oligonucleotides are printed on the arrays and the use of multiple short sequences (base mismatch strategy in the center of an additional partner sequence) help to increase specificity and reproducibility. However, oligonucleotide microarrays are much more expensive than cDNA microarrays because specialized expensive equipment -for hybridization, labelling, washing, and analysis- is needed. Also, sensitivity can be affected, but multiple probes can solve that problem 14.
DNA microarray applications
DNA microarrays are useful to analyze the transcripts of cancer cells making a gene expression comparison with normal cells. The data obtained from the DNA microarray images can be used to identify patterns with similar transcriptional activity and therefore to group together genes that are related with cell proliferative state. In other words transcript profiling and clustering are power tools for sub-classification of tumor types which may lead to a better diagnosis and therapy of cancer 16. Besides, important studies have been made in mitosis and meiosis -an analysis of chances in gene expression at different times- of budding yeast. Cho et al. used synchronized cells at a homogenous cell-cycle state while Spellman et al. used an asynchronous vegetative culture. In the first case 416 periodic transcripts were identified whereas in the Spellman et al. experiment 800 periodic transcripts were observed 16. These examples show another potential application of DNA microarrays in the study of periodic cell-cycle genes of other organisms.
Another interesting application of DNA microarrays is in the field of stress response and aging, gene expression of cells varies depending on the environmental conditions by which are surrounded. Human myeloid cells have been analyzed after being treated with genotoxic agents and ionizing radiation and unsuspected genes were expressed as a result 18. In this study, many cellular responses took place such as DNA metabolism, signal transduction and cell-cycle control 16.
In another study calf muscles of young and old mice (stress of age) were evaluated and 113 genes were observed 19, many of them related with energy metabolism, protein turnover, stress response and other pathways. What was found in this study is that caloric restriction (increases lifespan) prevent the transcription many of the 113 genes 16. These studies are some applications for DNA microarrays, however this technology has advanced in such a way that another review paper is required to explain the known and the new DNA microarray applications that are still been discovered.
Data analysis
Next to the step of macromolecular hybridization between the specific samples of labeled DNA with the DNA probes continues the data obtained analysis process to finally result in the interpretation of results. To carry out this analysis, the microarray plate is scanned by a scanning confocal microscope with the objective of producing data in the form of images that will later be transmuted into numeric data sets 2. In order to make this numerical information meaningful, in the context of results interpretation, the obtained sets of numerical data are «normalized» 4. That is, the data sets are parameterized to obtain systematic differences among them. As mentioned by Terca 4 a clear example of normalization is the reforming of dye intensity values to compensate for the efficiency of DNA markers in a dual-channel microarray experiment where labels that reflect two different colors have been used. After normalization, a statistical analysis is needed by softwares such as ICEP or Nexus Expression then clustering and classification methods are applied 5.
However data analysis tends to be complicated because of large amounts of data that microarrays provide; specific algorithms have been developed by the scientific community to guarantee quality control 20. In each sample (data point) of a microarray it is possible to find up to 450,000 gene probes (variables). For this reason, it is essential to remove features that are irrelevant and redundant to reduce the dimensionality of the data; the problem with high dimensionality is that more information is required and the computational cost is higher. Then when there is many variables and a small number of samples, large datasets can have overfitting that lead to an increase in noisy features and classification errors. Noisy data can increase complexity in the proposed models and reduce the efficiency of machine learning algorithms. However, to solve the high dimensionality problem, these techniques are usually applied: feature subset selection and feature extraction 21.
Feature selection methods which remove trivial features (e.g. gene probes that only have low levels of activity), are now required due to the size of the data generated in these last years. They differ from feature extraction methods because they do not change the normal representation of the data 21. Besides, these methods are based on feature selection algorithms which are divided in three groups: filters, wrappers, and embedded techniques 21,22. The filters do not use any classifier and remove features without the use of any learning techniques contrary to wrappers which use those techniques to appraise useful features 21. Furthermore, embedded techniques usually use learning techniques to make selections that depend on specific classifier algorithms 22. This technique tends to be more computationally efficient than wrappers which are usually computationally inefficient, however the most efficient in this aspect are the filters 21.
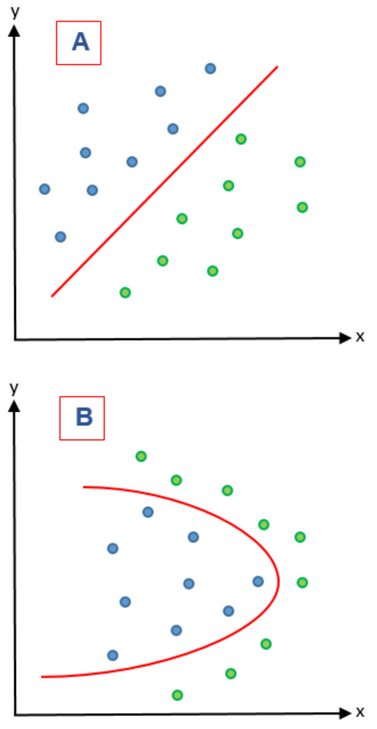
Figure 3: A) represents a linear problem while B) represents a nonlinear problem.
On the other hand, feature extraction methods combine variables in order to give a new variable and therefore reducing the dimensionality of the features that were combined. These methods are based on feature extraction algorithms are divided in two groups: linear and nonlinear. In Figure 3 these two kinds of problems are represented. In a linear feature extraction (LFE) the reduction of the dimensionality is done by a linear matrix factorization on a dimensional linear subspace that is lower. Instead, nonlinear feature extraction (NFE) has diverse ways to perform the dimensionality reduction; it can find nonlinear relationships among features on a low and high dimensional space 21. In a LFE, principal component analysis (PCA) is a widely used extraction algorithm 23 and is complemented by supervised principal component analysis to guarantee that the principal components of the dataset belong to the class variable 24. In NFE, lifting functions, kernel functions, Isomap algorithms (manifolds) and Kernel PCA have been used 21.
We have seen that feature selection and feature extraction are useful techniques to reduce irrelevant features, precisely to decrease the dimensionality of them. However there some advantages and disadvantages between these two techniques. Feature selection maintain the characteristics of the data, in other words, the data preserve its normal representation for interpretability. But the problem with this technique is that it has a low discriminative power and a reducing overfitting. On the other hand, feature extraction has a high discriminative power and can control overfitting, but the characteristics of the data can change and its transformation could be more expensive 21. After this feature selection or feature extraction to improve and prepare the data, it can be classified and clustered by classifiers 25 and various software such as CLIC, FIGS, SEURAT, AutoSOME, ConsensusCluster, GcExplorer and so forth 5.
Microarray databases
As a result of the analysis and monitoring of data obtained by some of the types of DNA microarray we have the generation of an enormous sequence of more data that must be conserved in two possible ways: a) through the use of specific software that fulfill the role of a database on a local scale and to store the data obtained on-site, and b) databases that act as mass storage of public information. In this case, it is necessary to take into account three aspects necessary for the dissemination of data coming from the microarray, which are: 1) follow standard parameters for data collection, 2) adhere to standard parameters established for the exchange of data, and 3) use the public information stores kindred to DDBJ, EMBL, and GenBank. Minimum Information About Microarray Experiment (MIAME) and Microarray Gene Expression Markup Language (MAGE-ML). MIAME is the standard format for data collection and MAGE-ML is the file format based on XML data exchange which was grown-up by Microarray Gene Expression Data (MGED) society and Object Management Group (OMG) 3,26. As reference, Table 1 shows a bibliographic compilation that contains the most available microarray databases which follow the data dissemination parameters mentioned above.
Table I: Bibliographic compilation of microarray databases
CONCLUSIONS
Microarrays are emerging technologies in the field of biotechnology whose development and refinement has been concentrated in the last two decades. By allowing the simultaneous and efficient monitoring of the gene expression of thousands of genes, it has come to occupy the functional role of classical techniques such as southern and northern blotting. The technical progress of DNA microarray technology since its inception in 1995 has allowed it to be considered as a strategic tool in the clinical field of medical diagnosis. The theoretical scheme of this method is based on the interaction of various elements (target, labels, probe, and solid support) among themselves applying the biological principle of macromolecular complementarity.
The use of cDNA or oligonucleotide microarrays will depend on the resources, equipment and the time available for the researcher. cDNA microarrays have a better sensitivity, lower cost, and the equipment needed are normally present in a laboratory while oligonucleotide arrays have a better specificity, less contamination, reproducibility is easier and the generation of the array is faster. However, cDNA microarrays require more time to prepare the probe; synthesis, purification and storage of DNA solutions requires more work and contamination could be a problem. Another problem is the specificity because cross hybridization can occur. Likewise, oligonucleotide microarrays are more expensive than cDNA microarrays (specialized equipment is needed to prepare and analyze the microarrays) and not all researchers and their laboratories can afford that. Sensitivity could also be a problem if multiple probes are not used.
One of the most important steps in the quantitative monitoring of gene expression is data analysis. This process involves the transmutation of data in form of images to numeric data sets. After this and following processes that include the parameterization of data, biologically meaningful information is obtained that can finally be analyzed, interpreted and presented in the form of results. Finally, all the data obtained from the data analysis are stored in databases according to standardized parameters and formats related to data collection and exchange of results.
The adequate preparation of DNA microarrays, its analysis and to store the information obtained from it in databases make possible a better understanding of cellular processes (DNA metabolism, signal transduction, cell-cycle, etc.). This is used to study cancer, mitosis and meiosis, cellular responses to stress (changes in the environment), infections, diseases and so forth, precisely to find better mechanisms for diagnosis and treatments in the medical field. DNA microarrays have been improved these last decades that is why this technology has many applications, some of them which are still been discovered.
REFERENCES
1. NHGRI. An Overview of the Human Genome Project [Internet]. National Human Genome Research Institute (NHGRI). 2017 [cited 12 August 2017]. Available from: https://www.genome.gov/12011238/an-overview-of-the-human-genome-project/
2. Xiang C, Chen Y. cDNA microarray technology and its applications. 2000.
3. Heller M. DNA Microarray Technology: Devices, Systems, and Applications. 2002.
4. Tarca A, Romero R, Draghici S. Analysis of microarray experiments of gene expression profiling. 2006.
5. Naidu C, Suneetha Y. Review Article: Current Knowledge on Microarray Technology – An Overview. Tropical Journal of Pharmaceutical Research. 2012;11(1).
6. Chalah A. Fundamentals of Microfluidics with Applications in Biological Analysis and Discovery. Harvard Extension School. 2016.
7. Ekins R, Chu F. Microarrays: their origins and applications. Trends in Biotechnology. 1999;17(6):217-218.
8. Russell S, Meadows L, Russell R. Microarray technology in practice. 2009
9. Sobek J, Bartscherer K, Jacob A, Hoheisel J, Angenendt P. Microarray Technology as a Universal Tool for High-Throughput Analysis of Biological Systems. Combinatorial Chemistry & High Throughput Screening. 2006;9(5):365-380.
10. Aguado M. DNA Microarrays: Principles and Technologies. 2012.
11. Kuo W, Whipple M, Jenssen T, Todd R, Epstein J, Ohno l. Microarrays and clinical dentistry. Journal Of The American Dental Association. 2003;4(134):456-62.
12. Giorgi F, Bolger A, Lohse M, Usadel B. Algorithm-driven Artifacts in median polish summarization of Microarray data. BMC Bioinformatics. 2010;11(1):553.
13. Leung Y, Cavalieri D. Fundamentals of cDNA microarray data analysis. 2003
14. Gundogdu O, Elmi A. Genome Resource Facility [Internet]. Grf.lshtm.ac.uk. [cited 12 August 2017]. Available from: http://grf.lshtm.ac.uk/microarrayoverview.htm
15. Conway T, K. G. Microarray expression profiling: capturing a genome-wide portrait of the transcriptome. Molecular Microbiology. 2003;47(4):879-889.
16. Epstein C. Microarray technology — enhanced versatility, persistent challenge. Current Opinion in Biotechnology. 2000;11(1):36-41.
17. Merck KGaA. Water for DNA Microarrays | Application | Water Purification | Merck [Internet]. Merckmillipore.com. 2017 [cited 12 August 2017]. Available from: http://www.merckmillipore.com/INTL/en/learning-centers%2Fapplications%2Fnucleic-acids%2Fdna-microarrays%2FdeGb.qB.3pgAAAFAfZkQWTdw,nav?ReferrerURL=https %3A%2F%2Fwww.google.com.ec%2F
18. Amundson S, Bittner M, Chen Y, Trent J, Meltzer P, Fornace A. Fluorescent cDNA microarray hybridization reveals complexity and heterogeneity of cellular genotoxic stress responses. Oncogene. 1999;18(24):3666-3672
19. Lee C. Gene Expression Profile of Aging and Its Retardation by Caloric Restriction. 1999.
20. Eijssen L, Jaillard M, Adriaens M, Gaj S, de Groot P, Muller M et al. User-friendly solutions for microarray quality control and pre-processing on ArrayAnalysis.org. Nucleic Acids Research. 2013;41(W1):W71-W76.
21. Hira Z, Gillies D. A Review of Feature Selection and Feature Extraction Methods Applied on Microarray Data. Advances in Bioinformatics. 2015;2015:1-13.
22. Bolón-Canedo V, Sánchez-Maroño N, Alonso-Betanzos A, Benítez J, Herrera F. A review of microarray datasets and applied feature selection methods. Information Sciences. 2014;282:111-135.
23. Shlens, J. A Tutorial on Principal Component Analysis. 2014
24. Barshan E, Ghodsi A, Azimifar Z, Zolghadri Jahromi M. Supervised principal component analysis: Visualization, classification and regression on subspaces and submanifolds. Pattern Recognition. 2011;44(7):1357-1371.
25. Widodo A, Yang B. Application of nonlinear feature extraction and support vector machines for fault diagnosis of induction motors. Expert Systems with Applications. 2007;33(1):241-250.
26. Penkett C, Bähler J. Navigating Public Microarray Databases. 2004.
Received: 12 June 2017
Approved: 10 august 2017
All pictures used in this article belong to the authors:
1Henry J. Herrera and 1Marlon Gancino
1School of Biological Sciences and Engineering
Yachay Tech
Hacienda San José s/n Urcuquí, Ecuador
E-mail: henry.herrera@yachaytech.edu.ec and marlon.gancino@yachaytech.edu.ec
Yachay Tech
Hacienda San José s/n Urcuquí, Ecuador
E-mail: henry.herrera@yachaytech.edu.ec and marlon.gancino@yachaytech.edu.ec